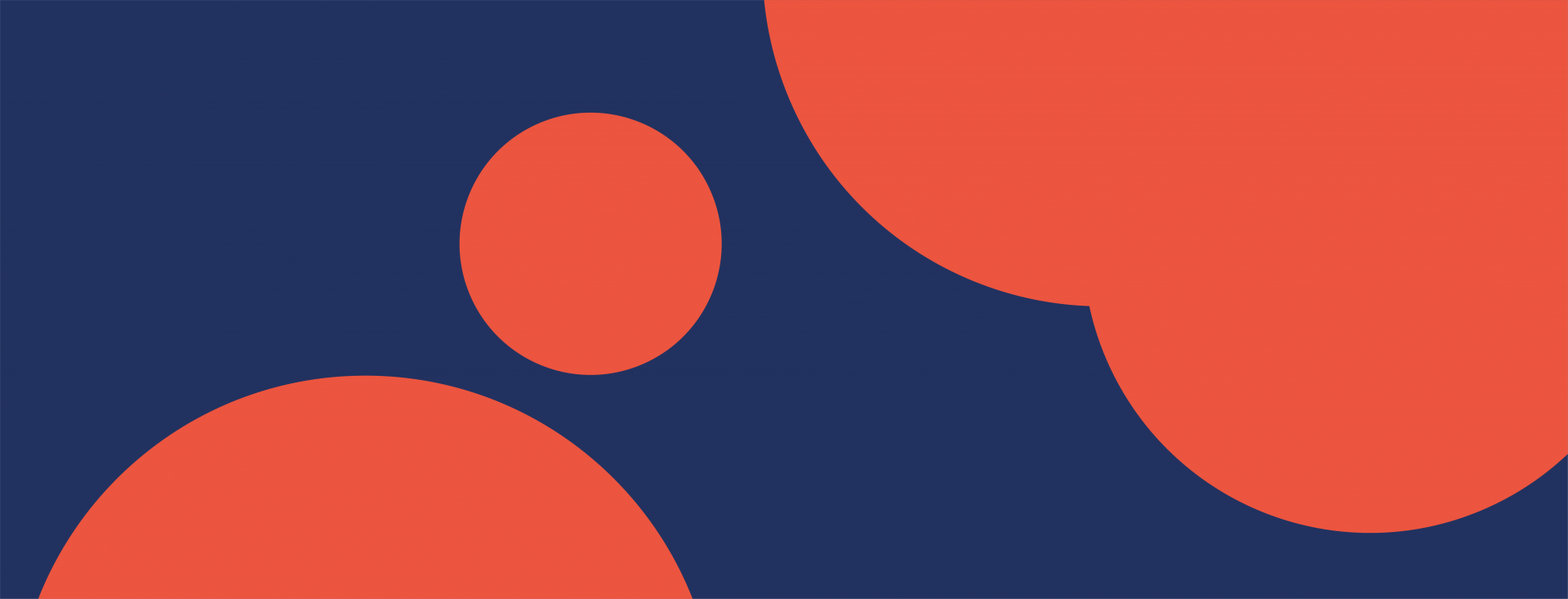
6 Questions (and Answers) with Tony Jebara, VP of Machine Learning
Tony Jebara, Spotify’s new Vice President of Machine Learning, says he started studying the algorithm-based technology when he was in college, “before it was cool.” Now, machine learning is not only undeniably cool, but it’s also incredibly practical—it also enables fan-favorite playlists like Discover Weekly, and more recent creations like On Repeat and Repeat Rewind.
Tony and his team of engineers and research scientists, therefore, have a two-fold mission: To analyze data on what users search and stream, and use those learnings to run experiments that turn into some of your favorite personalized playlists and personalized homepages.
We recently sat down with Tony, and he explained why, after four years as the director of machine learning at Netflix, he was intrigued by Spotify, where machine learning is central to our company strategy.
As a guitarist and songwriter, it was a perfect—dare we say algorithmic—fit.
First, what is machine learning? How do you use it at Spotify?
Machine learning finds patterns in data in a statistically reliable way so that we are confident they were not flukes. Then, it studies that data to determine what actions to take for each context in order to maximize reward. We’re not just trying to find patterns in the data, but cause and effect relationships too.
At Spotify, machine learning helps us match millions of users to the content (e.g. tracks, podcasts) most relevant to them at an unparalleled speed. We’re aiming to facilitate the user journey and make it enjoyable so that it doesn’t involve as much hunting around on our app. It’s a way for us to say ‘you’re going to love these things, let me put them at the top of your page for you,’ and also accelerate that process based on what people with similar interests have discovered.
You came from Netflix, which is a really interesting player in the machine learning space. How does your work today leverage past experience?
There are lots of similarities. Both services have to algorithmically match users to the right content and both have to decide how to invest in content and creators. But one key difference is that the Spotify catalog is huge—there are over 50 million songs and hundreds of thousands of podcasts. On the flip side, the Netflix catalog only has to deal with thousands of movies and TV shows. So, machine learning and algorithms play a much more crucial role at Spotify.
What makes Spotify’s application of machine learning unique or special?
If you think about what Spotify does, we deliver really, truly personalized experiences on a global level and in localized markets. Creating one personalized playlist for one user in one market can be challenging, but it’s doable with a human curator. We take cultural aspects into consideration, because in culture it’s about more than drawing a straight line from the past into the future. Cultural shifts are sometimes erratic or anything but linear. That’s why we increasingly invest in systems that combine human experts and algorithms. While humans are good at articulating the “new, interesting and unexpected twist”, algorithms are better at scaling that curation to a personal experience for millions of people.
If you have a catalog of millions of songs and a global market of, you know, 200 million plus, you need to be able to scale your efforts thoughtfully. Machine learning allows and enables us to do that at the speed and quality consistency that Spotify is known for.
Our algorithms allow us to scale out very personalized, hand-selected experiences that help members feel were made just for them. The goal is to deliver an amazing listening experience.
What will machine learning mean for our creators—artists and podcasters—on platform?
With machine learning, we can expand our audience analytics capabilities in a way that helps creators get new fans. It’s no longer just about knowing if your song has been downloaded or streamed 8 million times, it’s about creating a connection between artists, creators and their fans. With machine learning, we can actually start to inform them about what types of people are consuming their work, at what time, and what it gets consumed with. You know—like pairing wine with food. What songs are this podcast dining well with? Things like that help unclog creative potential because people can understand their audiences better. Then they get valuable feedback, something that so many creators crave.
Machine learning is a fast-moving field, to say the least. What do you think the future of machine learning will look like? Let’s say three years from now?
Over the next three years, machine learning will become more causal and long-term. Right now, machine learning mostly uncovers superficial input-output relationships. For example, given what you played today, here’s what you’ll play tomorrow. This leads to short-term engagement but might not yield long-term satisfaction. My hope is that three years from now, machine learning becomes less myopic. It should figure out the best sequence of actions to lead you on a journey where you discover new great audio content, become more engaged, and stay satisfied as a listener for years to come.
Now before we go, are there any podcasts you’re especially into right now? When do you listen to them?
I’m kind of nerdy, so I like Stuff You Should Know. It’s not a story, it’s just interesting things around nutrition or technology or political facts. I like to listen to it and learn about some random new things popping up.
I usually listen to podcasts while I’m lying in bed, when I don’t want to hold my screen or have the blue light keep me up but still want to learn something new.