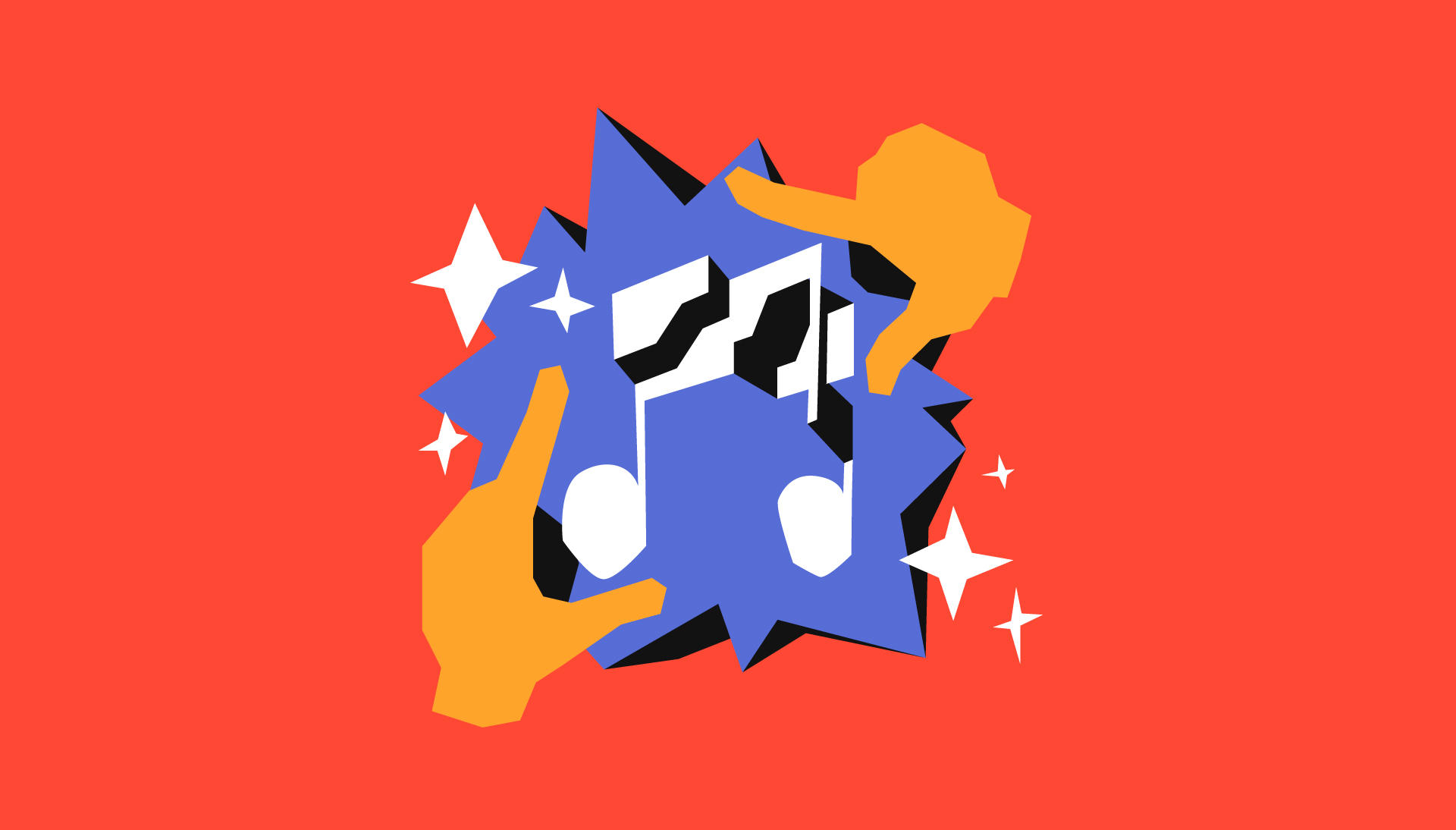
Every day, teams across Spotify leverage AI and machine learning to apply our personalization capabilities on a large scale, leading to the features, playlists, and experiences Spotify users have come to know and love. And when you spend your days working with emerging technologies, it’s easy to get transfixed by complicated new advancements and opportunities. So how do our forward-thinking teams ensure they can tackle this technical work while also prioritizing the experience of our users?
That’s a question constantly on the mind of Emily Galloway, Spotify’s Head of Product Design for Personalization. Her team’s role is to design content experiences that connect listeners and creators. This requires understanding our machine learning capabilities as they relate to personalization to leverage them in a way that is engaging, simple, and fun for our users.
“Design is often associated with how something looks. Yet when designing for content experiences, we have to consider both the pixels and decibels. It’s more about how it works and how it makes you feel,” Emily explains to For the Record. “It’s about being thoughtful and intentional—in a human way—about how we create our product. I am a design thinker and a human-centric thinker at my core. People come to Spotify to be entertained, relaxed, pumped up, and informed. They come for the content. And my team is really there to think about that user desire for personalized content. What are we recommending, when, and why?”
The Personalization Design team helps create core surfaces like Home and Search, along with much-loved features like Discover Weekly, Blend, and DJ. So to better understand just how to think about the design behind each of these, we asked Emily a few questions of our own.
How does design thinking work to help us keep our listeners in mind?
When you work for a company, you know too much about how things work, which means you are not the end user. Design helps us solve problems by thinking within their mindset. It’s our job to be empathetic to our users. We have to put ourselves in their shoes and think about how they experience something in their everyday life. A big thing to keep in mind is that when using Spotify, phones are often in pockets and people look at the screen in quick, split-second moments.
Without design, the question often becomes, “How do we do something technically?” For those of us working at Spotify, we understand how or why we’re programming something technically in a certain way, but users don’t understand that—nor should they have to. What they need is to experience the product positively, to get something out of it. We’re accountable for creating user value. We really are there to keep the human, the end user, at the forefront.
Without this thinking, our products would be overcomplicated. Things would be confusing and hard to use, from a functionality perspective. Good design is about simplicity and should largely remain invisible.
But design is also additive: It adds delight. That’s what I love about projects like DJ or Jam that are actually creating connection and meaning. Design is not afraid to talk about the emotional side—how things make you feel.
How does design relate to personalization?
Personalization is at the heart of what we do, and design plays an important role in personalization.
Historically, Spotify’s personalization efforts happened across playlists and surfaces like Home and Search. But over time we utilized new technologies to drive more opportunities for personalization. This started from a Hack Week project back in the day to become Discover Weekly, our first successful algorithmically driven playlist. It then gave way to Blend, which was designed for a more social listening experience. And more recently, to DJ, our new experience that harnesses the power of AI and editorial expertise to help tell artists’ stories and better contextualize their songs. It utilizes an AI voice that makes personalization possible like never before—and it’s a whole new way for our listeners to experience Spotify’s personalization.
When designing personalized experiences like these, we must think “content first,” knowing people come to Spotify for the content. Design ultimately makes it feel simple and human and creates experiences that users love. If recommendations are a math problem, then resonance is a design problem.
But we also have to have what I like to call “tech empathy”—empathy for the technology itself. My team, which is a mix of product designers and content designers, has to understand how the technology works to design our recommendations for the programming. Personalization designers need to understand the ways in which we’re working with complex technology like machine learning, generative AI, and algorithms. Our designers need to consider what signals we’re getting that will allow our recommendations to get better in real time and overtime. And when a recommendation is wrong, or a user just wants a different mood, we need to design mechanisms for feedback and control. That really came into play when we developed our AI DJ.
Tell us the story of the inception of DJ.
We’re always trying to create more meaningful connections between listeners and creators in new and engaging ways. And we use technology to deliver this value. DJ is the perfect example of how we’re driving deeper, more meaningful connections through technology.
Prior to generative AI, a “trusted friend DJ” would have required thousands of writers, voice actors, and producers to pull this off—something that wasn’t technically, logistically, or financially possible. Now, new technologies have unlocked quality at scale. Xavier “X” Jernigan’s voice and personality delivers on our mission of creating more meaningful connections to hundreds of millions of people. Generative AI made the once impossible feel magical.
To bring DJ to life we answered some core experiential questions knowing we are taking listeners on a journey with both familiar and unfamiliar music. We asked questions such as: What does it mean to give context to listening? How do we visualize AI in a human way? You can see this in how the DJ introduces itself in a playful way—owning that it’s an AI that doesn’t set timers or turn on lights.
We also put a lot of thought into how we designed the character, since it is more than a voice.
Ultimately, we really wanted to lean into making it feel more like a trusted music guide, as well as having an approachable personality. So much of our brand is human playfulness, so we made a major decision to acquire Sonantic and create a more realistic, friendly voice. And that led to Xavier training the model to be our first voice. His background and expertise made him the perfect choice.
With new technologies like generative AI, what are some of the new ways you’re thinking about your team and their work?
I’m challenging our team to think differently about the intersection of design and generative AI. We keep coming back to the conclusion that we don’t need to design that differently because our first principles still stand true. For example, we are still taking a content-first approach and we continue to strive for clarity and trust. We’ve realized that tech advancements are accelerating faster than ever, which makes design’s role more important than ever.
Because there’s so much more complexity out there with generative AI, it means the human needs must be kept in mind even more. At the end of the day, if our users aren’t interested in a product or they don’t want to use it, what did we create it for?
Emerging technology inspires you to think differently and to look from different angles. The world is trying to figure this out together, and at Spotify we’re not using technology to use technology. We’re using technology to deliver joy and value and meet our goals of driving discovery and connections in the process.
Recent Comments